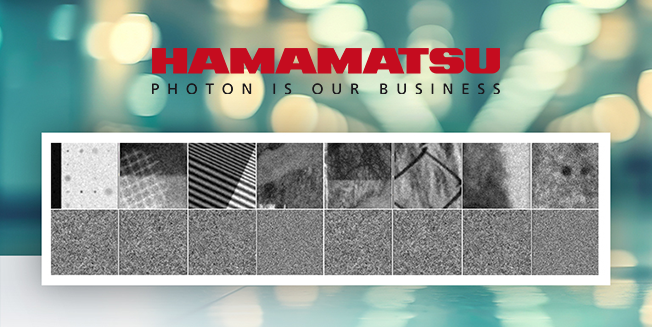
Deep learning is a method of artificial intelligence (AI) that mimics how humans think and learn, allowing computers to learn by example. At its core are ‘artificial neural networks’, computational models based on neural interconnections of the human brain. Deep learning is constructed upon several hidden layers of neural networks that perform complex operations: each layer calculates the values given as input to the next layer, elaborating the information more and more exhaustively.
In deep learning, a computer model can master classification tasks, when provided with sufficient data, directly receiving images as input, i.e. recognizing cats in animal photos. Nonetheless, it can learn how to discriminate noise from structural parts of an X-ray image.
Training the AI is the most challenging part of deep learning: a large amount of computational power is required, together with a vast data set. In the specific case of X-ray image de-noising, when learning from measured images, over tens of thousands of images can be necessary to reach the required performance, which is a significant drawback when using this method.